Conférenciers invités
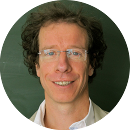 |
|
High Dimensional Learning and Deep Neural Networks
Stéphane MALLAT
Professeur Ecole Normale Supérieure, Paris Membre de l’Académie des Sciences
|
|
|
Abstract: Classification and regression require to approximate functions in high dimensional spaces. Avoiding the dimensionality curse opens many questions in statistics, probability, harmonic analysis and geometry. Recently, convolutional deep neural networks have obtained spectacular results for image analysis, speech understanding, natural languages and many other problems. We shall describe their architecture and analyze their mathematical properties, with many open questions. These architectures implement multiscale contractions, where wavelets have an important role. Applications will be shown for image and audio classification, and for regressions of molecular energies in quantum chemistry. |
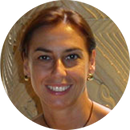 |
|
Clustering evolving multiple data streams
Rosanna VERDE
Professeur Seconda Università di Napoli - Italy
|
|
|
Abstract: In this talk we introduce a new strategy for clustering data streams and for monitoring their evolution over time. Our proposal is based on a first summarization of sub-sequences though histograms. Then, a two phase strategy is performed: a partition of the sub-sequences of non-overlapping time windows in order to get a further summarization of streams and an updating of a dissimilarity matrix; a final clustering analysis on the dissimilarity matrix of the local partitions in order to obtain a final streams partition. The data streams changing is detected by considering the evolution of a partition of the streams over a user chosen time period and the evolutions in proximity relations. The summary of the sub-sequences by histograms allows to evaluate changing owed to the several components (location, size and shape) of sub-sequence distributions over the time. That is due to the use of the Wasserstein distance (in 2-norm) to compare distributions that can be decomposed in two components related to the different characteristics of the distributions. Through an application on real and simulated data, we will show that this method provides results comparable to algorithms for stored data. |
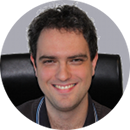 |
|
Metric Learning with fewer Constraints
Amaury HABRARD
Professeur Université Jean Monnet, Saint-Etienne Laboratoire Hubert Curien
|
|
|
Abstract: Distance metrics play a crucial role in many machine learning and data mining algorithms. Optimizing a metric for a task at hand is then of high importance to the performance of these algorithms. In this context, a lot of approaches have focused on learning Mahalanobis-like distances mostly by using similarity or dissimilarity constraints between examples. However, taking into account all these constraints can be prohibitive for some tasks when the number of examples increases. In fact, we will show that it is possible to learn a Mahalanobis distance by using a linear set of constraints consisting in moving learning examples to a priori defined virtual points. This approach admits a close form solution that can be easily kernelized. Furthermore, it has some consistency guarantees and we will show that it is as expressive as methods based on pairwise constraints learning algorithms. We will also provide two solutions for defining the so-called virtual points: an easy one using class label information and a second based on recent works in optimal transport. |
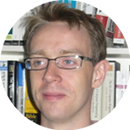 |
|
Véracité des Big Data
Pierre SENELLART
Professeur Télécom ParisTech Département Informatique et Réseaux
|
|
|
Abstract: Le Big Data est souvent défini par 4 « v » : volume, vélocité, variété, véracité. Ce dernier, cependant, est rarement pris en compte dans les applications. Dans cet exposé, nous présentons une vue d'ensemble de deux domaines de recherche lié à l'estimation et l'exploitation de la véracité des données. Nous traitons tout d'abord de la détection de la vérité (ou truth finding), c'est-à-dire des techniques de fouille de données exploitant les corrélations entre données et sources d'information, grâce à des analyses statistiques et des algorithmes à point fixe, pour estimer la véracité en l'information. Nous évoquerons ensuite le problème de la gestion de l'information incertaine à grande échelle, en présentant en particulier des techniques permettant d'effectuer le calcul efficace de la probabilité d'une information résultant d'un calcul sur un grand volume de données. |
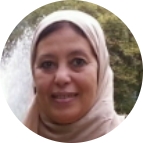 |
|
Modélisation et simulation de la croissance d’une tumeur
Rajae ABOULAICH
Professeur EMI Rabat, Maroc LERMA
|
|
|
Abstract: Comprendre complètement comment une tumeur cancéreuse se développe demeure un enjeu important en oncologie. Plusieurs approches sont utilisées pour modéliser et simuler l’évolution d’une tumeur, parmi lesquelles les modèles mathématiques. Ces modèles peuvent être utilisés à différent échelles dans le but de décrire et de simuler le phénomène: à l’échelle moléculaire, cellulaire, tissulaire ou à l’échelle des organes en prenant en compte les échanges entre les différentes parties du corps. Les modèles mathématiques sont continus ou discrets, via des équations ou des agents, l’objectif étant de comprendre et de simuler l’évolution des cellules et de la tumeur. La dynamique des cellules tumorales, leurs interactions les uns avec les autres et avec leur environnement, le transfert de substances chimiques et de nombreux phénomènes peuvent être représentés et étudiés grâce à des abstractions mathématiques. Notre objectif est de présenter dans un premier temps un modèle continu utilisant un problème de contrôle avec comme contraintes des EDP par lequel nous cherchons à simuler l’évolution d’une tumeur. Dans la deuxième partie nous présentons une approche Hybride utilisant la modélisation multi-agents couplée aux équations aux dérivées partielles. |
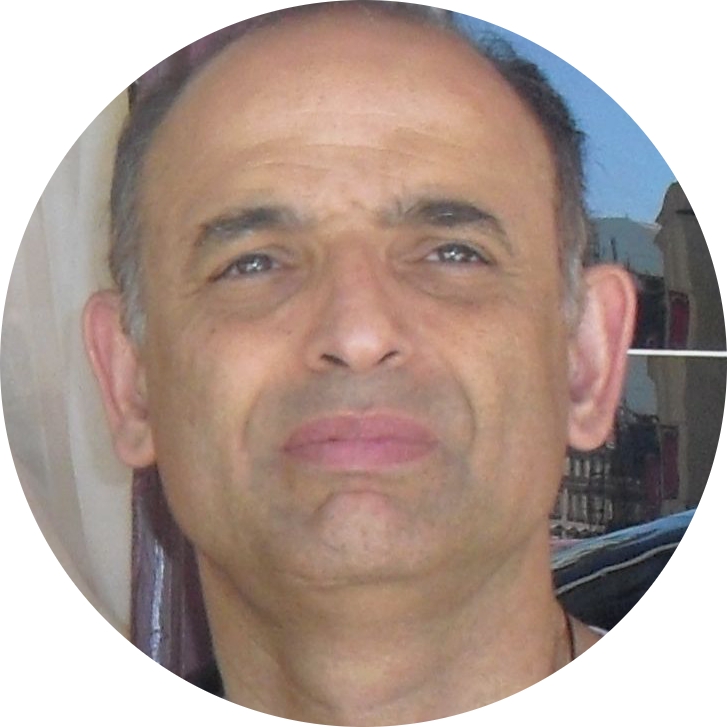 |
|
Numerical Simulations of Complex Fluid Flows
Fayssal BENKHALDOUN
Professeur Université Paris 13 - Sorbonne Paris Cité LAGA-CNRS
|
|
|
Abstract: The objective of this presentation is to introduce a survey on adaptive finite volume methods for complex computational fluid dynamics. Mathematical modelling of fluid dynamics in flow systems is based on the formulation and numerical solution of appropriate equations of conservation of mass, momentum and energy. In most realistic applications analytical solutions of these models do not exist and numerical methods are therefore required for their simulations and consequently understanding their dynamics. Irregular domains and complex input data are among the difficulties encountered when designing numerical techniques for this class of application in modern technology. However, with the high improvements achieved in the computer resources, high computing performance could be a key in simulating these challenging problems with reasonable accuracy using personal computers and laptops. As a matter of fact, developing an adaptive finite volume black-box has became one of the worldwide active research fields in computational fluid dynamics. In this survey we present a review on recent advances in adaptive finite volume methods for industrial applications. More precisely, we discuss the application of this class of methods for the following situations: • Shallow water problems • Streamer propagation in cold Plasma • Flows in porous media
|
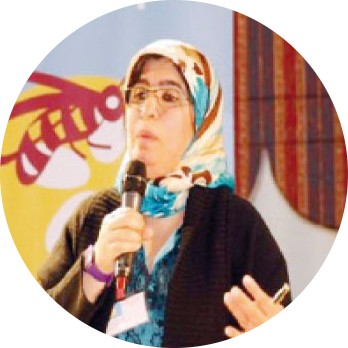 |
|
No Bees No Life
Badiâa LYOUSSI
Professeure USMBA - Fès Head of Laboratory Physiology - Pharmacology - Environmental Health
|
|
|
Abstract: “The fact is that of the 100 crop species that provide 90 per cent of the world’s food, over 70 are pollinated by bees.”- UN report. If the bees became extinct, the plants that depend on bees for pollination will die. Wheat, barley, rye, rice, bamboo and corn are pollinated solely by the wind. Butterflies are also one of the major pollinators in addition to birds. Pears can be pollinated by hand after the bees go extinct, just like in southern Sichuan. This costly labor intensive hand pollinating processwould bring hundreds of jobs where people would dip feathered sticks into pollen and dab each individual flower on each individual tree.
|
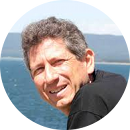 |
|
Tutoriel 1 : L’induction : un saut à l’élastique. Mais avec quel élastique ?
Antoine CORNUÉJOLS
Professeur Agro ParisTech Dept. Modélisation Mathématique, Informatique et Physique
|
|
|
Abstract: Hume, en 1737, affirmait qu’il n’y a pas de base absolue sur laquelle fonder l’induction. Le thème du tutoriel sera d’examiner différents principes imaginés en apprentissage automatique pour permettre le « saut inductif ». On s’attachera également à en percevoir la généalogie, et donc les évolutions futures imaginables, une sorte de méta-induction.
Le plan prévu est le suivant : - Qu’est-ce que l’induction ? Quelles en sont les formes ? - Les principes inductifs dans l’histoire de l’apprentissage automatique : quels types de sauts inductifs ? quelles performances cherche-t-on à mesurer ? quelles théories sous-jacentes ? - Le principe de minimisation du risque empirique et ses descendants - L’apprentissage par transfert va t-il nous conduire à inventer d’autres formes de principes inductifs ? - Induction et éducation, et autres perspectives
|
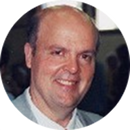 |
|
Tutoriel 2 : Classification non supervisée et modèles de mélange
Gérard GOVAERT
Professeur Université de Technologie de Compiègne Heudiasyc
|
|
|
Plan du tutoriel : 1. Classication non supervisée Objectif Exemples Approches classiques Critère d'inertie intra-classe CAH de Ward Algorithme des centres-mobiles Problèmes posés 2. Démarche probabiliste en classication non supervisée Introduction Cadre général Approches non-paramétriques Approches paramétriques 3. Le modèle de mélange Introduction Le modèle Exemples Estimation des paramètres Algorithme EM 4. Utilisation du modèle de mélange en classication Les deux approches La vraisemblance classiante L'algorithme CEM Comparaison de deux approches Lien avec la classication floue 5. Exemple du mélange gaussien multivarié Dénition Les algorithmes EM et CEM Modèle parcimonieux Algorithmes associés aux modèles parcimonieux 6. Mise en ÷uvre de la classication à base de mélange Sélection de modèles (a) Introduction (b) Critères de sélection (c) Illustrations numériques Stratégie d'initialisation et règle d'arrêt Prise en compte de situations particulières (a) Données spatiales (b) Données manquantes (c) Données bruitées (d) Apprentissage semi-supervisé 7. Classication croisée avec des modèles probabilistes Classication croisée Modèle des blocs latents Les deux approches Conclusion 8. Les logiciels
|
|